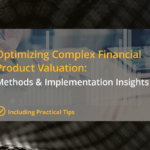
Optimizing Complex Financial Product Valuation
28. January 2025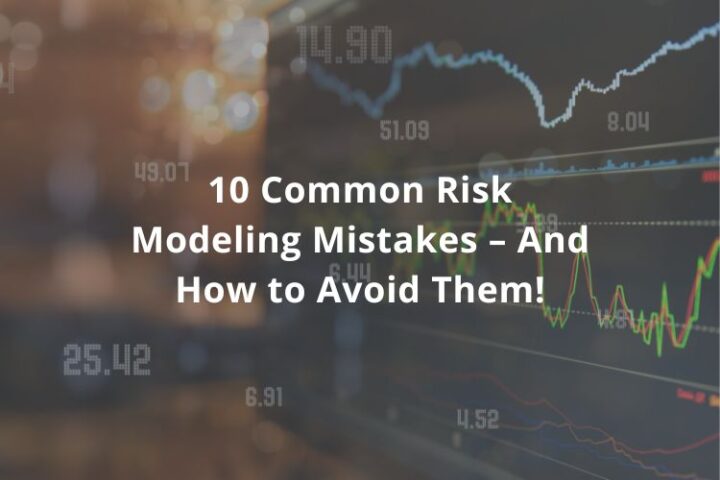
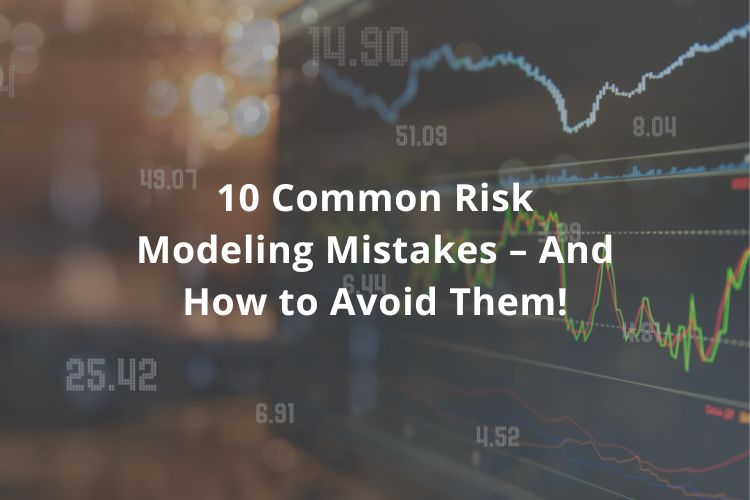
10 Common Risk Modeling Mistakes – And How to Avoid Them
Risk models are central to financial decision-making and regulatory compliance. Yet even sophisticated frameworks can fail – not because of complex mathematics, but due to avoidable assumptions, oversights, or misapplications. In this article, we outline ten common risk modeling mistakes observed across the financial industry, along with actionable strategies to improve resilience and integrity.
1. Using Outdated or Inaccurate Market Data
Your model is only as good as the data it consumes. Stale or poorly transformed inputs skew forecasts and undermine capital assessments.
How to avoid it: Use timely, validated data sources. Implement automated cleansing and reconciliation routines to detect inconsistencies before they cascade through the model.
2. Ignoring Key Risk Factors
Oversimplification in the name of usability can mean omitting critical risk drivers – especially under stress.
How to avoid it: Leverage sensitivity analysis and machine learning-based validation to identify hidden dependencies. Engage subject matter experts to sense-check exclusions.
3. Ignore Extreme Events (Fat Tails)
Standard risk measures (e.g., Value at Risk – VaR) often fail to capture rare but severe market shocks. Value at Risk remains widespread but offers little insight into tail risk.
How to avoid it: Complement VaR with Expected Shortfall (ES) and stress testing. Regulatory frameworks like Basel IV are already pushing toward ES adoption.
4. Underestimating Correlation Effects
Many models assume correlations between assets remain constant, even in crisis situations. Correlations behave well in calm markets – and collapse under stress. Assuming otherwise can severely misprice portfolio risk.
Example: During the 2008 financial crisis, asset correlations spiked, amplifying risk instead of mitigating it.
How to avoid it: Use dynamic correlation models and stress-aware copulas to better reflect shifting dependencies during volatility regimes.
5. Using Inappropriate Volatility Models
Traditional models underestimate market dynamics. Volatility isn’t a constant – it clusters, spikes, and evolves. Relying on fixed assumptions underestimates real exposure.
How to avoid it: Apply GARCH or stochastic volatility models, calibrated to current market behavior. These are standard in quant trading – and increasingly relevant to risk teams.
6. Ignoring Model Risk
Relying on a single model without validation can create a false sense of accuracy and hide structural weaknesses. No single model captures reality. Without cross-validation, blind spots go unnoticed.
How to avoid it: Benchmark results across independent approaches. Use challenger models to test assumptions and expose structural weaknesses.
7. Poor Calibration of Model Parameters
Models don’t fail because they’re wrong – they fail because they’re outdated. Using outdated parameters or failing to recalibrate models regularly can lead to significant deviations from market reality.
How to avoid it: Recalibrate frequently. Use parameter drift alerts and review windows tied to market shifts, not just calendar schedules.
8. Misinterpreting Model Outputs
Numbers alone don’t tell the full story. Blindly trusting results without considering the broader context leads to poor decisions and missed red flags.
How to avoid it: Use contextual interpretation frameworks, peer benchmarks, and expert oversight to ensure consistent analysis.
9. Inadequate Stress Testing
Stress scenarios are often too mild or entirely missing, failing to reveal true vulnerabilities. Treating stress testing as a box-checking exercise defeats its purpose. Many scenarios remain too narrow or too tame.
How to avoid it: Build reverse stress tests and calibrate scenarios using real crisis data. Simulate impact beyond capital metrics – think liquidity, reputational damage, and contagion.
10. Overlooking Regulatory Requirements
Non-compliance with the latest risk regulations can lead to severe penalties. Models must evolve with regulatory expectations. Static compliance introduces exposure.
How to avoid it: Maintain a compliance matrix linking models to current rules. Include documentation, sign-offs, and review cycles as part of model governance.
Conclusion
Risk models are decision tools – not answers. Their value lies in how well they reflect uncertainty, adapt to change, and hold up under scrutiny. Avoiding these ten mistakes isn’t about perfection – it’s about vigilance, challenge, and continuous learning.
Want to build better models? Learn how UnRısk supports model validation, scenario design, and regulatory alignment! Book a strategy call.